At first glance, Franz Kafka and the modern digital world may seem different universes. If we examine Kafka’s The Metamorphosis closely and the evolution of identity fraud, we find similarities in the transformation and depersonalisation of identity.
In The Metamorphosis, Gregor Samsa wakes up one day converted into an insect, a radical change that alters his perception of himself and how others see him. Similarly, the evolution of identity fraud involves a metamorphosis in how individuals are seen in the digital world. Digital identity can be manipulated and distorted, leading to a loss of control over one’s image, like in deepfakes.
In Kafka’s literary work, Gregor’s new form causes ambiguity in his identity and purpose in the world. In the digital sphere, identity becomes equally ambiguous, as cybercriminals who perform such frauds can create fake profiles or use stolen information to blur the lines between the authentic and the fake.
The protagonists face a confusing and often incomprehensible reality in The Metamorphosis and digital identity fraud. Gregor struggles to adapt to his new form. At the same time, victims of this type of fraud often face difficulty understanding how the impersonation happened and what steps they must take to restore their identity.
Nevertheless, this threat endangers the victims’ civil status and the companies that see their processes and systems breached.
This article dives into the art of addressing the new challenges of identity fraud, providing not only a clear picture of the problem but also possible solutions and investigations to detect fraud and protect the integrity and security of users. From datasets for a robust deepfake detection system through recent research to advanced authentication measures.
But first, let’s take a look at the evolution of this type of fraud.
The evolution of identity fraud
In the beginning, identity fraud was based on the simple usurpation of physical documents such as passports and ID cards. Fraudsters use techniques such as pickpocketing or ID card manipulation to get personal information from their victims. Once under control of these documents, they were able to perform fraudulent transactions or even commit crimes in the name of the victim.
With the improvement of technology, identity fraud evolved to exploit vulnerabilities in computer systems and communication networks. It gave rise to what is now known as “cyber identity fraud“. Criminals began to use social engineering to steal data from databases to obtain sensitive information such as passwords, credit card numbers and national insurance numbers. Furthermore, the emergence of techniques such as spoofing attacks and the use of deepfakes add additional layers of complexity to identity fraud, allowing fraudsters to manipulate information and create fake content more convincingly.
The proliferation of social networks and the information available online has provided fraudsters with an additional source of personal data. They can collect information about people’s activities, interests and relationships through social media profiles, allowing them to create fake profiles more convincingly.
Another noteworthy development is the rise of identity theft for financial fraud. Criminals can open bank accounts or apply for loans using the victim’s personal information, which can have serious financial and legal consequences for the person concerned.
New opportunities for digital identity fraud
Advances in technology have also opened up new opportunities for digital identity fraud. The widespread adoption of biometric technologies, such as facial recognition, has led to an increase in impersonation attempts using reverse engineering methods. Fraudsters are looking for ways to avoid these high-tech security systems, which poses an additional challenge for digital identity protection.
Furthermore, synthetic identity fraud, which combines elements of real and fake information to create a completely fictitious identity, represents a growing threat. Also, SIM Swapping, where attackers persuade mobile phone providers to transfer the victim’s phone number to a SIM card controlled by the attacker, provides access to text messages and calls, bypassing Two-Factor Authentication (2FA) and gaining access to digital accounts.
Detection of deepfakes
With the advent of various deep learning architectures, remarkable advances have been made in the field of image and video forgery. It has resulted in a notable increase in the production of fake multimedia content, driven by more accessibility and lower training requirements. Not only has the amount of such content grown, but also its level of sophistication has improved. It seems like it sometimes becomes indistinguishable from real videos.
An example of this happened in the 2020 Delhi elections, when a deepfake video of a popular politician was created, which is estimated to have been viewed by around 15 million citizens. Given the abuse and potential impact of deepfakes, more effective and robust detection methods are essential.
Data set to detect deepfakes
Designing a reliable deepfake detection system requires access to extensive deepfake datasets for training.
Table 1 lists the main features of publicly available deepfake datasets. Most of these datasets contain high-resolution images with a single face in the picture, although some include deepfakes generated with multiple generation techniques and multiple levels of compression. Nowadays, most content is shared via the web and social media, so it is common for videos and images to be shared in low resolution for more efficient transmission. We are increasingly seeing cases of fake videos in unrestricted environments, such as occlusions on the face (like glasses, hats, turbans or hijabs) and multiple faces with pose variations.
Table 1. Quantitative comparison of DF-Platter with existing Deepfake datasets
Note. Narayan, Agarwal, Thakral, Surbhi, Vatsa, and Singh (2023, p. 2).
So far, existing datasets have mainly consisted of single-subject deepfakes generated using a single technique. Nevertheless, it is also feasible to create deepfakes with multiple faked subjects. Recently, the developers at Collider released a deepfake with multiple fake faces in a single frame. The video, entitled “Deepfake Roundtable“, features a discussion between deepfakes of five famous personalities.
DF-Platter and KoDF
Datasets such as DF-Platter and KoDF play a crucial role in deepfake detection, serving as essential tools for training and evaluating deepfake detection algorithms, providing a variety of examples representing different techniques and scenarios, allowing detection models to learn to recognise deepfakes in a wide range of situations.
Furthermore, these datasets can cover deepfakes with occlusions (such as glasses, hats, and so forth.) and other challenges that complicate detection. It is essential for training models that can recognise deepfakes even in adverse conditions. In addition, they are often accompanied by annotations that provide information on the techniques used, the quality of the counterfeit and, in some cases, additional contextual information.
Finally, they allow the performance of detection algorithms to be assessed under realistic and diverse conditions, which is vital to ensure that the models are robust and effective in real-world scenarios.
Nevertheless, as counterfeits become more and more realistic, it becomes increasingly difficult to detect subtle and local traces of counterfeiting.
New approaches to presentation attack detection
As identity theft technologies become more sophisticated, detection constantly demands new approaches. The following methods are based on how to make artificial intelligence capable of generalising and adapting to detect unknown attacks. It is important to note that this is research and will possibly mark the future of more advanced identity fraud detection.
FLIP: Cross-domain Face Anti-spoofing with Language Guidance
What is it about?
FLIP is a new approach to face anti-spoofing (FAS) in face recognition systems, which uses contrastive language-image pre-training (CLIP) to improve generalisation in cross-domain anti-spoofing detection.
How does it work?
It is based on aligning visual representations with natural language class descriptions, which improves generalisability in low-data regimes. It includes three variants: FLIP-Vision (FLIP-V), FLIP-Image-Text Similarity (FLIP-IT) and FLIP-Multimodal-Contrastive-Learning (FLIP-MCL):
- FLIP-V is the simplest variant of FLIP and uses the base ViT model to detect facial presentation attacks. In this variant, the model is trained on a training dataset that includes images of real people and facial presentation attacks. The model learns to distinguish between genuine images and facial presentation attacks by comparing the image representations of the training images.
- FLIP-IT is another variant of FLIP that uses natural language supervision to generate more generalisable representations. In this variant, the model is trained on a training dataset that includes images of real people and facial presentation attacks, as well as text descriptions of the pictures. The model learns to distinguish between genuine images and facial presentation attacks by comparing the image and text representations of the training images.
- FLIP-MCL is the most advanced variant and uses a multimodal contrastive learning strategy to generate more generalisable representations. In this variant, the model is trained on a training dataset that includes images of real people and facial presentation attacks, as well as text descriptions of the pictures. The model learns to distinguish between genuine images and facial presentation attacks by comparing the image and text representations of the training images and uses a multimodal contrastive learning strategy to improve the generalisation ability of the model.
These variants use different strategies, such as aligning image representation with text and multimodal contrastive learning, to improve the detection of presentation attacks.
How does it differ from more traditional detection techniques?
Unlike traditional FAS approaches, which often have limited generalisation to unseen spoof types, camera sensors and environmental conditions, FLIP improves generalisation for the FAS task by using multimodal pre-training and alignment with natural language. It makes it more effective in cross-domain and data-limited situations.
Implicit Identity Driven Deepfake Face Swapping Detection
What is it about?
The IID approach focuses on the identification of explicit and implicit identities in facial images. In the context of face swapping, clear identity refers to the visible appearance of the face, while implicit identity encompasses underlying information crucial for the detection of deepfakes.
How does it work?
The IID framework uses a combination of explicit identity contrast loss (EIC) and implicit identity exploration (IIE) to differentiate between real and fake samples in the feature space, improving the detection of deepfakes and face swapping.
How does it differ from more traditional detection techniques?
Unlike other methods, which generally focus only on explicit identities (visible appearance of the face), IID also considers implicit identities, thus addressing the limitations of traditional methods in detecting more sophisticated forgery techniques. This approach has not only proven to be effective in detecting face swapping but also offers robust generalisation across multiple datasets, demonstrating its ability to adapt to different types of forgeries.
Dynamic Graph Learning with Content-guided Spatial-Frequency Relation Reasoning for Deepfake Detection
What is it about?
The SFDG method addresses the limitations of previous approaches in integrating frequency information with image content by using dynamic graph learning to analyse spatial and frequency features. We can say that it develops a graph model to exploit the relationships of the spatial and frequency domains to detect subtle clues of forgery.
How does it work?
This method consists of three main modules: Content-guided Adaptive Frequency Extraction (CAFE), Multiple Domains Attention Map Learning (MDAML) and Dynamic Graph-based Spatial-Frequency Feature Fusion Network (DG-SF3Net).
The way it works is as follows: the CAFE module extracts content-adapted frequency tracks, which allows the identification of specific frequency features that are difficult to spoof. The MDAML module enriches the spatial frequency contextual characteristics with multiscale attention maps, which helps to detect more complex tampering patterns. Finally, the DG-SF3Net explores the higher-order relationship between spatial and frequency features through graph convolution and channel-node interaction, allowing more subtle and sophisticated manipulations to be detected.
How does it differ from more traditional detection techniques?
The proposed dynamic graph method has several advantages over existing deepfake detection methods. First, the SFDG approach extracts content-adapted frequency cues, which allows for the identification of specific frequency features that are difficult to spoof. Secondly, the method uses dynamic graphs to fuse spatial and freq
ID-unaware deepfake detection model
What is it about?
It is a methodology designed to reduce the influence of the phenomenon known as “Implicit Identity Leakage” in the detection of deepfakes. This phenomenon refers to the tendency of binary classifiers to learn unexpected identity representations in images, which can impair their generalisation ability.
How does it work?
This model uses an Artifact Detection Module (ADM) and a Multi-scale Facial Swap (MFS) method. The ADM detects areas of artefacts in fake images using multi-scale anchors, which helps to focus on local regions of the images and reduces attention to global identity information. MFS, on the other hand, manipulates dummy and original images to generate new dummy images with position annotations of artefact areas, thus enriching the artefact features in the training set.
How does it differ from more traditional detection methods?
This model focuses on detecting local artefacts and reducing the dependency on global identity information, which improves its generalisability and effectiveness in detecting deepfakes.
Instance-Aware Domain Generalization (IADG)
What is it about?
IADG is a framework for identity fraud detection that focuses on domain generalisation by aligning features at the instance level. It seeks to weaken the sensitivity of components to specific instance styles to improve generalisation in unseen scenarios.
How does it work?
It includes three components: AIAW, which adaptively removes style-sensitive feature correlation; DKG, which generates instance-adaptive filters; and CSA, which creates style-diversified samples for each instance. These components work together to improve the generalisability of the model.
How does this differ from more traditional detection methods?
Current approaches typically make use of domain labels and focus on domain-level alignment. In contrast, IADG operates at the instance level, allowing for more effective generalisation in a variety of unseen scenarios.
Categorical Style Assembly (CSA)
What is it about?
CSA is a component of the IADG framework that generates samples with diversified styles to simulate style changes at the instance level.
How does it work?
This method considers the diversity of samples from various sources to generate new styles in a wider feature space. In addition, it introduces the categorical concept into the FAS task and separately augments real and fake samples to prevent negative label changes between different classes.
How does it differ from more traditional detection techniques?
Unlike traditional approaches that might mix font styles without considering frequency or categorical information, CSA generates styles in a more diversified and contextualised way, which improves the model’s ability to handle wider and more complex style variations.
Dynamic Kernel Generator (DKG)
What is it about?
DKG is a component of the IADG framework that focuses on the automatic generation of instance-adaptive filters, which is key to domain generalisation.
How does it work?
DKG uses a dynamic filtering approach to adapt to the diversity of samples in multiple source domains. It facilitates the learning of adaptive features to instances, which is crucial for generalisation across different contexts and types of attacks.
How is it different from more traditional detection methods?
Unlike traditional methods that use static filters, DKG adapts to specific features.
FAS-wrapper
What is it about?
DKG is a component of the IADG framework that focuses on the automatic generation of instance-adaptive filters, which is key to domain generalisation.
How does it work?
DKG uses a dynamic filtering approach to adapt to the diversity of samples in multiple source domains. It facilitates the learning of adaptive features to instances, which is crucial for generalisation across different contexts and types of attacks.
How is it different from more traditional detection methods?
Unlike traditional methods, the FAS wrapper handles the problem of updating models in different domains without the need to retrain the FAS model from scratch completely. In conventional approaches, adaptation to new domains often involves extensive retraining with data from the new domain, which can be costly in terms of resources and time. In addition, these traditional methods often suffer from catastrophic forgetting, where the acquisition of new knowledge results in the loss of previously learned knowledge.
Asymmetric Instance Adaptive Whitening (AIAW)
What is it about?
AIAW focuses on domain generalisation in facial spoofing detection by asymmetrically adapting the correlation of style-sensitive features for each instance to improve generalisation.
How does it work?
This method selectively suppresses sensitive covariance and highlights insensitive covariance. It uses instance normalisation to produce a normalised feature and then calculates the covariance matrix of this feature. Different selective ratios are applied to suppress sensitive covariance in real and fake faces.
How is it different from more traditional detection techniques?
What makes AIAW different from other approaches is that it does not rely on artificial domain labels and focuses on instance-level domain generalisation. It makes it more effective in adapting to instance-specific variations and in improving generalisation in unseen scenarios.
Advanced authentication measures: biometrics and liveness detection
Advanced authentication measures, in particular biometrics and liveness detection, represent a significant change in the way identity is verified in the digital environment. Biometrics, which is based on the unique physical features of an individual, is considered to be one of the most secure authentication methods. This biometric approach offers an additional layer of security, as biological features are inherently more difficult to replicate or forge compared to traditional passwords.
On the other hand, there is liveness detection, a complementary technique to biometrics that analyses the vitality of a person in a digital process. This method seeks to confirm that the user present in front of the system is a real person and not an attack. The user can be asked to perform certain facial movements such as blinking, gestures, changes in expression, and eye movements, among others (active liveness detection), to achieve it. Technology providers such as Mobbeel also perform what is known as passive liveness detection. Unlike active measures, where the user performs specific actions to demonstrate their physical presence, passive liveness detection is performed continuously, analysing a micro video of the user without the user having to perform additional actions.
These indicators and analyses are difficult for an automated system to replicate by an impostor or through the use of fake images or videos.
The future: technological innovation and adaptability for fraud prevention
The future of digital security is shaping up to be an environment where adaptability and technological innovation will be key to addressing the emerging challenges of identity fraud. In the near future, the integration of advanced technologies and proactive strategies will become the standard to ensure digital identity protection.
One of the prominent trends focuses on the development of smarter and more adaptive authentication systems. These systems move away from traditional password-only authentication towards a multi-factor approach that combines multiple layers of verification. The fusion of biometrics, liveness detection and machine learning will enable the creation of a more robust and dynamic security ecosystem.
Artificial intelligence (AI) and machine learning will play a crucial role in detecting and preventing identity fraud. AI algorithms are becoming increasingly sophisticated, capable of analysing large volumes of data to identify patterns and anomalies that may indicate possible fraud attempts. The trend seems to be towards domain adaptation and generalisability. To achieve this adaptability, the research discussed above has focused on developing techniques that allow models to learn continuously and dynamically, constantly updating their understanding of what constitutes normal and abnormal behaviour. The challenge lies in how to teach AI to recognise subtle patterns or variations that might indicate a new form of attack, even in situations where no historical data is available.
Furthermore, more convergence is expected between biometric technologies and the Internet of Things (IoT), enabling more contextual authentication. Everyday devices, from smartphones to connected home devices, could integrate biometric authentication systems to ensure more secure and transparent identification in different scenarios and environments.
Data privacy and security will be a primary focus in this evolution. The implementation of stricter data protection standards and the adoption of privacy-by-design practices will be essential to ensure that the collection and use of biometric information is done in an ethical and privacy-compliant manner.
Contact us if you would like to incorporate a state-of-the-art identity verification system capable of detecting identity fraud.
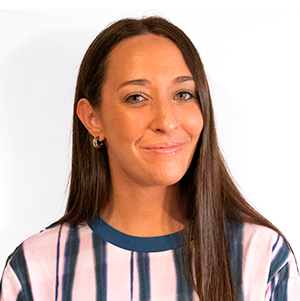
I am a curious mind with knowledge of laws, marketing, and business. A words alchemist, deeply in love with neuromarketing and copywriting, who helps Mobbeel to keep growing.
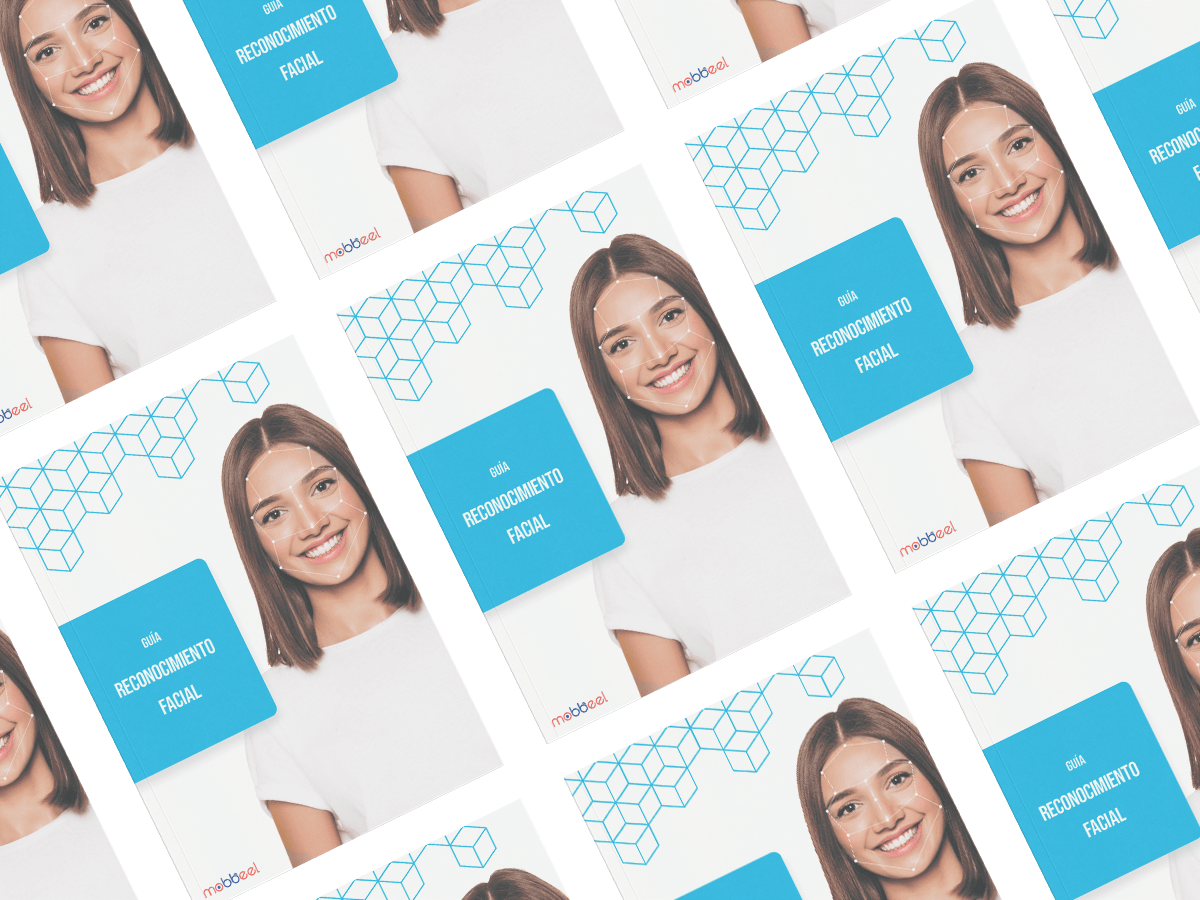
GUIDE
Identify your users through their face
In this analogue-digital duality, one of the processes that remains essential for ensuring security is identity verification through facial recognition. The face, being the mirror of the soul, provides a unique defence against fraud, adding reliability to the identification process.